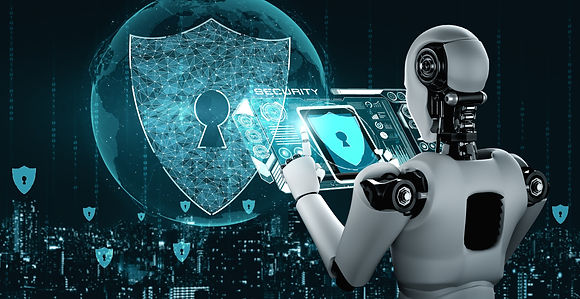
Next-Gen Cybersecurity:
The AI Revolution
COURSE OBJECTIVE
Advanced course on the use of artificial intelligence to strengthen the cybersecurity of banks. The XOI methodology for measuring cyber risk is briefly explained.
The global vision of CyberRisk, cyberattacks and losses suffered by financial institutions, methodologies and good practices on cybersecurity in business processes are exposed, and some technical standards for management and control are explained, such as NIST, Cobit 5. and ISO 27001.
Cyber Risk Appetite, Cyber Risk Limits and Cyber Risk Tolerance methodologies for the governance and control of Cyber Risk are exposed.
Traditional methodologies such as logistic regression and other, innovative, machine learning methodologies are exposed, such as: decision trees, naive bayes, KKN, LASSO logistic regression, random forest, neural networks, Bayesian networks, Support Vector Machines, gradient boosting tree, etc
The use of artificial intelligence and in particular machine learning and deep learning to strengthen cybersecurity is explained, advanced models are shown to detect anomalies, transactional fraud, phishing, cyber attacks, intrusions and malware.
There are four modules dedicated to advanced deep learning, that of convolved neural networks for facial recognition, the Generative Adversarial Network (GAN) to detect adverse attacks from machine learning algorithms, recurrent neural networks for the classification of CyberRisk events, and multilayer. perceptron for intrusion and anomaly detection.
The exercises have been developed in two powerful languages, Python and R, and are presented in the JupyterLab environment to enhance learning.
WHO SHOULD ATTEND?
This program is aimed at directors, managers, consultants, regulators, auditors and risk analysts, operational risks, cyber risks, as well as those professionals who are implementing cybersecurity measures. Professionals who work in banks, savings banks and all those companies that are exposed to cyber risks. Statistical and mathematical knowledge is required.
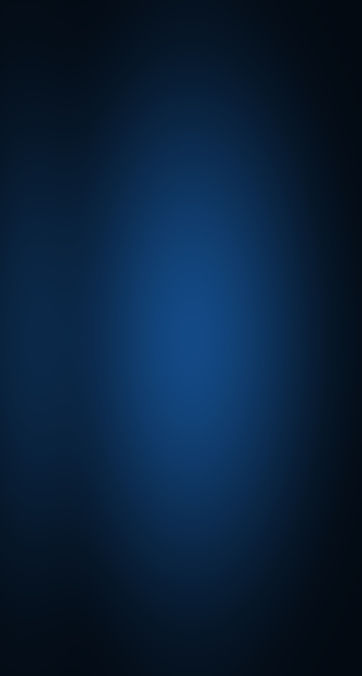


Price: 6.900 €
Schedules:
-
Europe: Mon-Fri, CEST 16-19 h
-
America: Mon-Fri, CDT 18-21 h
-
Asia: Mon-Fri, IST 18-21 h

Level: Advanced

Duration: 30 h

Material:
Presentations: PDF
Exersises: Python, Cython, R and Jupyterlab

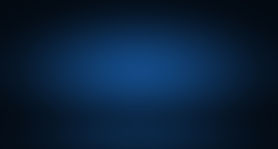
AGENDA
Next-Gen Cybersecurity:The AI Revolution
Cybersecurity in Basel III
Module 1: Cyber Resilience
-
Cyber risks in banking
-
CyberRisks in Latin America and Europe.
-
Cyber Resilience Standards and Guidelines
-
Case Study 1: Recent Regulatory Initiatives: Australia, Germany and the US Minimum Requirements
-
Cybergovernance
-
cybersecurity strategy
-
Management roles and responsibilities
-
Recognition of the importance of the board of directors and senior management
-
Variety of supervisory approaches regarding the second and third lines of defense (3LD)
-
Case Study 2: Roles and responsibilities of chief information officers (CISOs) in cyber governance
-
Cyber risk awareness culture
-
Architecture and standards
-
Cybersecurity Workforce
-
Case Study 3: Frameworks for professional cybersecurity training and certification programs
-
-
Risk management, testing, and incident response and recovery approaches
-
Methods for monitoring cyber resilience
-
Risk specialists assess information security management and controls
-
Jurisdictions are increasingly engaging with industry to address cyber resilience
-
-
Testing of information security controls and independent assurance
-
Mapping and classification of business services should inform testing and assurance
-
Penetration Test
-
Taxonomy of cyber risk controls
-
-
Response and recovery and exercise tests
-
Service continuity assessment, response and recovery plans, and continuous learning
-
Joint public-private exercise
-
Case Study 4: “Exercise Resilient Shield”
-
-
Cybersecurity and resilience metrics
-
Cybersecurity and resilience metrics
-
Emerging Resilience Indicators
-
-
-
Communication and information exchange
-
Overview of cross-jurisdictional information sharing frameworks
-
Sharing information between banks or peers
-
Case Study 5: FS-ISAC: key features and benefits
-
Sharing from banks to regulators
-
Sharing between regulators
-
Case Study 6 - Bilateral exchange of cybersecurity information between the Hong Kong Monetary Authority (HKMA) and the Monetary Authority of Singapore (MAS)
-
Module 2: Cyber Risks
-
Current Vision of Cyber Risk
-
identification of cyber risks
-
Malware and other threats
-
-
Cyber-Security in practice
-
Security Government
-
Risk management
-
Security politics
-
Safeguards Policy
-
Contingency Plans
-
-
Security Audits
-
Some Cyber risks
-
Exploit Kits
-
Information leakage
-
phishing
-
DDoS attack
-
internet of things
-
infrastructure attack
-
botnets
-
trojans
-
Advanced Malware
-
Ransomware
-
APT's
-
-
Avoidance, Acceptance, Mitigation or transfers of Cyber-Risks (Cyber-Insurance)
-
Incident Response
-
Legal Aspects of Incident Response
-
Computer forensics
-
Digital Compliance
-
Intelligence in Open Sources
-
Brand Defense. Intellectual Property Rights
-
Digital Reputation. Crisis Communication
-
Fraud and Online Identity Management
-
Computer Law and ICT's
-
Procedural Law and Human Rights in Cyber-Space
-
Penal responsibility of juridical persons
-
Cryptography and Authentication Systems
-
Industrial Cyber-Security (IT/OT)
-
Logic Cyber-Security
-
Assurance
-
Cyber risks in banking
-
financial robberies
-
Attacks on banking transactions
-
credit card theft
-
Wholesale Banking
-
Lazarus cyber attack on the SWIFT system
-
Bank resources allocated to cybersecurity
-
Cloud service provider (CSP)
-
Analysis of the main CSP providers
-
Analysis and duration of blackouts in the Cloud service
Module 3: Cyber Risk Management
-
Enterprise Risk Management in cyber risk
-
Involvement of senior management
-
Cybersecurity in business processes
-
Identification of:
-
Critical bank assets
-
critical business functions
-
Critical business partners: customers, suppliers, outsourcing
-
Critical data, critical connections
-
Main threats to the bank
-
Framework for Improving Critical Infrastructure Cybersecurity NIST
-
Main technical standards
-
NIST 800-53
-
Cobit 5
-
ISO 27001
-
-
Policies and control of cyber risk
-
Governance of Cyber Risk in practice
-
Lines of defense
-
risk analysis
-
Probability and impact maps
-
CyberRisk mitigation strategies
-
Identity and access management
-
data protection
-
Security analytics using machine learning
-
NIST CSF pillars
-
safety hygiene
-
Recovery time objective
-
Recovery point goal
-
SSDLC
-
Risk management of third-party technology
-
-
Security architecture
-
Cloud environment and mobile security
Module 4: Measuring Cyber Attacks using XOI Approach: Exposure, Occurrence and Impact
-
Exposure Definition
-
Selection of KRIs
-
Exposure modeling and conditioning
-
Definition of hypothesis
-
Modeling and conditioning of the occurrence
-
Occurrence quantification
-
Impact quantification
-
Types of indicators
-
Indicator predictability
-
Bayesian networks
-
The Bayesian Network Scenario Model
-
graphic interpretation
-
Simulation using Bayesian networks
-
XOI modeling for Cyber risks in banking
-
Cyber Risk Scenarios
-
Banking cyberattacks
-
Exercise 1: XOI modeling in cyberattacks using Bayesian networks in Python and R
Module 5: Cyber Risk Appetite
-
Principles of an effective Cyber Risk Appetite methodology
-
Definitions and analysis:
-
Risk appetite framework
-
Risk Appetite Statement
-
Risk Tolerance
-
Risk Capacity
-
Risk Profile
-
-
Establishment of Limits in Cyber Risk
-
Principles of Effectiveness of the Cyber Risk Appetite Statement
-
Establishment of Limits and Metrics in Cyber Risks
-
Establishment of risk limits and tolerance in CibeRiesgos
-
Incorporation of Cyber Risk Appetite in decision making, new products, new lines of business, etc.
-
Mitigation plans
Machine Learning for Cybersecurity
Module 6: Machine Learning
-
Artificial intelligence
-
Definition of Machine Learning
-
Machine Learning Methodology
-
Data Storage
-
Abstraction
-
Generalization
-
Assessment
-
-
Supervised Learning
-
Unsupervised Learning
-
Reinforcement Learning
-
Deep learning
-
Typology of Machine Learning algorithms
-
Steps to Implement an Algorithm
-
Information collection
-
Exploratory Analysis
-
Model Training
-
Model Evaluation
-
Model improvements
-
Machine Learning in consumer credit risk
-
-
Machine Learning in credit scoring models
-
Analysis of main tools: R, Python, Microsoft Azure, SAS Enterprise Miner, SAS Visual Analytics, Knime, IBM SPSS Modelller, Spark, etc.
Module 7: Artificial Intelligence AI for Cyber Security in Banking
-
AI artificial intelligence for cybersecurity
-
Detection of cyber security anomalies
-
Advanced deep learning models
-
-
Use of Supervised Learning in cybersecurity
-
Use of Unsupervised Learning in cybersecurity
-
Detection and mitigation of Phishing
-
SVM
-
clustering
-
-
Deep Learning for the detection of attacks and malware
-
Recurrent Neural Networks
-
-
Intrusion detection
-
Network Traffic Analysis
-
Botnet detection
-
Machine learning to detect DDoS attacks
-
Detection of fraud in financial transactions
-
Detection on sensors
-
Banking fraud analytics
-
Advanced machine learning techniques for cybersecurity
-
Main vendors of AI for cybersecurity
-
Visualization tools
Unsupervised Learning to detect anomalies
Module 8: Unsupervised models
-
Hierarchical Clusters
-
K Means
-
standard algorithm
-
Euclidean distance
-
Principal Component Analysis (PCA)
-
Advanced PCA Visualization
-
Eigenvectors and Eigenvalues
-
Exercise 2: Principal components in R and SAS
-
Exercise 3: Anomaly detection with K-Means R
Supervised Learning to detect Fraud and anomalies
Module 9: Logistic Regression and LASSO Regression
-
Transactional fraud detection models
-
Econometric Models
-
Logit regression
-
probit regression
-
Piecewise Regression
-
survival models
-
-
Machine Learning Models
-
Lasso Regression
-
Ridge Regression
-
-
Model Risk in Logistic Regression
-
Exercise 4: Fraud score Logistic Regression in SAS and R
-
Exercise 5: Fraud score Logistic Regression Lasso in R
Module 10: Trees, KNN and Naive Bayes
-
Decision Trees
-
Modeling
-
Advantages and disadvantages
-
Recursion and Partitioning Processes
-
Recursive partitioning tree
-
Pruning Decision tree
-
Conditional inference tree
-
Tree display
-
Measurement of decision tree prediction
-
CHAID model
-
Model C5.0
-
K-Nearest Neighbors KNN
-
modeling
-
Advantages and disadvantages
-
Euclidean distance
-
Distance Manhattan
-
-
K-value selection
-
Probabilistic Model: Naive Bayes
-
Naive bayes
-
Bayes' theorem
-
Laplace estimator
-
Classification with Naive Bayes
-
-
Probabilistic Model: Naive Bayes
-
Naive bayes
-
Bayes' theorem
-
Laplace estimator
-
Classification with Naive Bayes
-
Advantages and disadvantages
-
-
Exercise 6: Anomaly detection with R decision tree
-
Exercise 7: Detection of KNN anomalies in R and SAS
-
Exercise 8: Naive Bayes Anomaly Detection in R
Module 11: Support Vector Machine SVM
-
SVM with dummy variables
-
SVM
-
Optimal hyperplane
-
Support Vectors
-
Add costs
-
Advantages and disadvantages
-
SVM visualization
-
Tuning SVM
-
Kernel trick
-
Exercise 9: Detection of Support Vector Machine anomalies in R
-
Exercise 10: Fraud Score support Vector Machine in Python data 2
Module 12: Neural Networks (Neural Networks NN)
-
Artificial neuron
-
Perceptron Training
-
Perceptron
-
Backpropagation algorithm
-
Training procedures
-
Tuning NN
-
NN display
-
Advantages and disadvantages
-
Exercise 11: Fraud Score using Neural Networks: multilayer perceptron in R data 1
-
Exercise 12: Fraud Score Neural Networks in Python data 2
Module 14: Ensemble Learning for Phishing
-
Set models
-
Bagging
-
Bagging trees
-
Random Forest
-
Boosting
-
Adaboost
-
Gradient Boosting Trees
-
Advantages and disadvantages
-
Exercise 14: Phishing detection Boosting in R
-
Exercise 15: Phishing detection in R
-
Exercise 16: Random Forest phishing detection, R and Python
-
Exercise 17: Phishing Detection Gradient Boosting Trees
Module 15: Validation of Machine Learning models
-
Out of Sample and Out of time validation
-
Checking p-values in regressions
-
Validation of time series MSE, MAD
-
Waste diagnosis
-
cross validation
-
Error bootstrapping
-
Binary case confusion matrix
-
Multinomial case confusion matrix
-
ROC curve
-
confidence intervals
-
Jackknifing with discriminant power test
-
Bootstrapping with discriminant power test
-
Kappa statistic
-
K-Fold Cross Validation
-
Traffic Light Analysis
-
Exercise 18: K-Fold Cross Validation of machine learning and deep learning models in Python and R
-
Exercise 19: ROC curve estimation in Python and R
-
Exercise 20: Bootstrapping ROC in R
-
Exercise 21: Kappa estimation and multinomial and binary confusion matrix in Python and R
Supervised Learning to detect Fraud and anomalies
Module 16: Deep Learning
-
Definition and concept of deep learning
-
Why now the use of deep learning?
-
Neural network architectures
-
Activation function
-
Sigmoidal
-
Rectified linear unit
-
Hypertangent
-
Softmax
-
-
Cost function
-
Gradient descending optimization
-
Using Tensorflow
-
Using Tensorboard
-
R deep learning
-
Python deep learning
-
Use of deep learning
-
How many hidden layers?
-
How many neurons, 100, 1000?
-
How many times and size of the batch size?
-
What is the best activation function?
-
-
Deep Learning Software: Caffe, H20, Keras, Microsoft, Matlab, etc.
-
Deployment software: Nvidia and Cuda
-
Hardware, CPU, GPU and cloud environments
-
Deep Learning Typology
-
Feedforward neural network
-
Multilayer Perceptron
-
-
Convolutional Neural Networks
-
Use of deep learning in image classification
-
-
Recurrent neural networks
-
Temporal series
-
Long Short Term Memory
-
Module 17: Deep Learning Feed Forward Neural Networks for anomalies, intrusions and traffic analysis
-
Cyber risks intrusions
-
Network traffic analysis
-
Single Layer Perceptron
-
Multiple Layer Perceptron
-
Neural network architectures
-
Activation function
-
Sigmoidal
-
Rectified linear unit (Relu)
-
The U
-
Selu
-
Hyperbolic hypertangent
-
Softmax
-
Other
-
-
Back propagation
-
Directional derivatives
-
gradients
-
Jacobians
-
Chain rule
-
Optimization and local and global minima
-
-
Exercise 22: Time Series Anomaly Detection Using LSTM
-
Exercise 23: Intrusion detection using convolved neural networks
-
Exercise 24: Network traffic analysis using multilayer perceptron neural networks
Module 18: Deep Learning CNN Convolutional Neural Networks for Facial Recognition
-
Facial Recognition for cybersecurity
-
CNN for pictures
-
Design and architectures
-
convolution operation
-
descending gradient
-
filters
-
strider
-
padding
-
Subsampling
-
pooling
-
fully connected
-
Credit Scoring using CNN
-
Recent CNN studies applied to credit risk and scoring
-
Exercise 25: Facial Recognition using Deep Learning CNN
Módulo 19: Deep Learning Redes Neuronales Recurrentes RNN
-
Selección y clasificación de ciberriesgos
-
Natural Language Processing
-
Natural Language Processing (NLP) text classification
-
Long Term Short Term Memory (LSTM)
-
Hopfield
-
Bidirectional associative memory
-
Gradiente Descendiente
-
Metodos de optimización globales
-
RNN y LSTM en las finanzas
-
Modelos unidireccionales y bidireccionales
-
Deep Bidirectional Transformers for Language Understanding
-
BERT Google
-
-
Ejercicio 26: Deep Learning CNN vs RNN para la clasificación de documentos
-
Ejercicio 27: Credit Scoring usando Deep Learning LSTM
Módulo 20: Adversarial machine learning
-
Ataques adversos para la ciberseguridad
-
Tipología de ataques adversos
-
Muestras adversarias
-
Generative Adversarial Network (GAN)
-
Fast Gradient Sign Method (FGSM)
-
Creación de muestras de malware adversarial utilizando GAN
-
Ejercicio 28: Ataque basado en gradiente en clasificación de imagen errónea