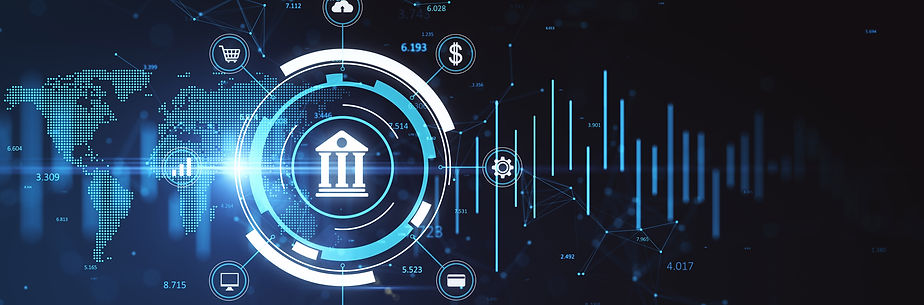
OBJETIVO DEL CURSO
Curso intensivo de metodologías de IFRS 9 de riesgo de crédito aplicando modelos tradicionales econométricos y de machine learning clásicos así como novedosos modelos de machine learning probabilístico, AI Generativa y computación cuántica.
La pandemia de COVID-19 surgió solo dos años después de la implementación en 2018 del IFRS 9. La pandemia estreso y afecto el poder predictivo de los modelos y metodologías, planteando desafíos significativos para la creación de provisiones de activos deteriorados. A raíz del shock pandémico, las posteriores medidas regulatorias y gubernamentales, así como el reciente conjunto, sin precedentes, de eventos de riesgo, tales como la guerra, la inseguridad en el suministro energético europeo y las presiones inflacionarias mundiales, los bancos han planeando gradualmente recalibrar los modelos de pérdida de crédito esperada (ECL) de la IFRS 9 para mejorar su precisión e incorporar las lecciones aprendidas. No obstante, aunque sean necesarios ajustes a los modelos, siguen apareciendo nuevos shocks macroeconómicos influidos por una elevada incertidumbre.
Las entidades han enfrentaron varios desafíos. El primero, fue el incremento significativo del riesgo de crédito (SICR) que se basó en información inexacta o incompleta. Segundo, la probabilidad de incumplimiento (PD) no era lo suficientemente sensible a la información prospectiva y no lineal. Tercero, los bancos aplicaron overlays con mayor frecuencia, pero no los justificaban ni cuantificaban.
Algunas firmas prestigiosas de consultoría proponen automatizar más procesos, desarrollar modelos desafiantes de PDs y pérdidas esperadas crediticias ECL.
Por lo anterior, hemos creado un curso, con un mayor número de modelos de estimación y calibración de la lifetime PD, hemos incrementado los modelos de inteligencia artificial y añadido modelos basado en algoritmos cuánticos que por un lado pueden ser modelos desafiantes de los tradicionales y que ayudarán a medir las relaciones no lineales.
No obstante, el core del curso es explicar pormenorizadamente metodologías de riesgo de crédito para estimar y calibrar los parámetros tipo lifetime de PD, LGD y EAD ajustados a la norma IFRS 9 empleando modelos econométricos, enfoque bayesiano, machine learning tradicional, machine learning cuántico y algortimos cuánticos.
Todos los modelos deben cuantificar la incertidumbre inherente a las inferencias y predicciones financieras para que sean útiles en la toma de decisiones y gestión de riesgos financieros. Los parámetros y resultados del modelo pueden tener un rango de valores con probabilidades asociadas. Por lo que se necesitan modelos probabilísticos matemáticamente sólidos que se adapten a las imprecisiones y que cuantifican las incertidumbres con coherencia lógica. Por lo anterior hemos incluido modelos de machine learning probabilístico, es decir algoritmos de aprendizaje automático junto con modelización probabilística y teoría de decisión bayesiana. Estos algoritmos ofrecen modernas y potentes soluciones en este complejo entorno financiero y económico de hoy en día.
Este curso incluye más de 12 metodologías y ejercicios para estimar la PD Lifetime en carteras de retail, hipotecas, pymes y corporate, por ejemplo, el modelo Exogenous Maturity Vintage EMV, modelos de Markov, modelos de supervivencia, matrices de transición, Deep Learning, algoritmos cuánticos de simulación de Monte Carlo entre otros.
Se han incorporado metodologías de forecasting y stress testing para generar escenarios económicos forward looking. Respecto al tema, hay varios módulos dedicados al diseño de escenarios donde se exponen la interacción entre las variables macroeconómicas y la Lifetime PD. Además, se explican metodologías de stress testing de las provisiones de riesgo crédito IFRS 9.
En cuanto a la LGD Lifetime, se muestran modelos de machine learning para mejorar la exactitud de los parámetros. Y respecto a la EAD Lifetime se explican modelos vintage para líneas de crédito, además de modelos econométricos de prepago.
Se entrega, una herramienta de pricing, que incluye la estimación del ECL 12m y ECL Lifetime, capital regulatorio, Raroc y Hurdle rate.
El Machine Learning cuántico es la integración de algoritmos cuánticos dentro de programas de Machine Learning. Los algoritmos de machine learning se utilizan para calcular inmensas cantidades de datos, el aprendizaje automático cuántico utiliza qubits y operaciones cuánticas o sistemas cuánticos especializados para mejorar la velocidad de cálculo y el almacenamiento de datos realizado por algoritmos en un programa. Por ejemplo, algunas técnicas matemáticas y numéricas de la física cuántica son aplicables al deep learning clásico. Una red neuronal cuántica tiene capacidades computacionales para disminuir la cantidad de pasos, los qubits utilizados y el tiempo de cómputo.
El objetivo del curso es mostrar el uso de la computación cuántica y redes tensoriales para mejorar el cálculo de algoritmos de machine learning.
Mostramos como los algoritmos cuánticos aceleran el cálculo de la simulación de Monte Carlo, la herramienta más potente para desarrollar modelos de riesgo crédito, representando una ventaja importante para el cálculo del capital económico, lifetime PD y creación de escenarios de stress testing.
El objetivo del curso es exponer modelos clásicos frente a modelos cuánticos, explicar los alcances, beneficios y oportunidades.
Se exponen técnicas de AI Generativa para desarrollar modelos de lifetime PD avanzados.
Para facilitar el aprendizaje la mayoría de macros se entregan en Jupyter Notebook, un entorno interactivo web de ejecución de código R y Python, donde se incluyen, vídeos, imágenes, formulas, etc. que ayudan al análisis y explicación de las metodologías.
¿QUIÉNES DEBEN ASISTIR?
Este programa está dirigido a responsables, analistas y consultores de riesgos que estén inmersos en el desarrollo, validación o auditoría de los modelos de riesgo crédito IFRS 9 o para todos aquellos interesados. Para la mejor comprensión de los temas es recomendable que el participante tenga conocimientos de estadística. Puedes beneficiarte de las tecnologías de computación cuántica sin necesidad de tener conocimientos de física cuántica.
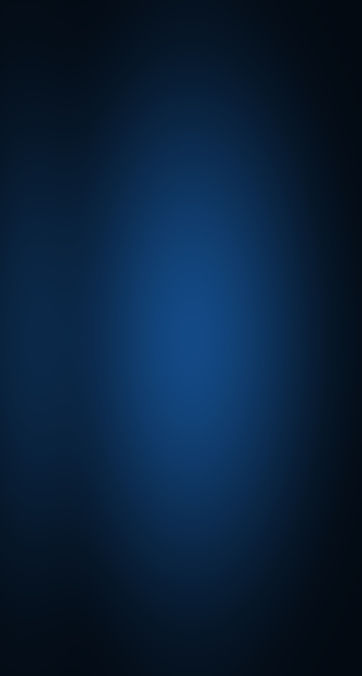

Horarios:
-
Santiago de Chile, Sao Paulo, Buenos Aires, Santo Domingo: L a V: 18-21h
-
España, Portugal: L a V 19-22 h
-
Ciudad de México, Quito, Bogotá, San José: L a V 19-22 h

Precio: 7 900 €

Nivel: Avanzado

Duración: 40 h

Material:
-
Presentaciones PDF
-
Ejercicios en Excel, R, Python y Jupyterlab
-
Se entrega el vídeo grabado del curso de 40 horas.
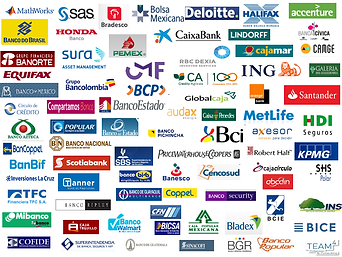

AGENDA
IFRS 9: Modelización del Riesgo Crédito 2.0
IFRS 9
Módulo 0: Implantación de la IFRS 9 en la UE
-
SICR assessment approaches
-
Approaches for determining stage transfers
-
Alignment between the Definition of Default and IFRS 9 exposures in Stage 3
-
Low credit risk exemption
-
12-month PD as proxy for lifetime PD
-
-
Expected Credit Loss Models
-
Types of Expected Credit Loss models
-
Model limitations and use of overlays
-
Effects from the Russian/Ukrainian conflict
-
ESG including climate risks
-
-
IFRS 9 PD variability and robustness
-
Variability in the IFRS 9 PD
-
Differences in the use of IRB models for IFRS 9 estimates
-
Differences in the definition of default
-
Differences in risk differentiation
-
Differences in risk quantification
-
Treatment of 2020-2021 defaults
-
-
Incorporation of forward-looking information
-
Macroeconomic scenarios
-
Variability of the methodological approach for incorporation of FLI and
reflection of non-linearity-
Incorporation of FLI at parameter level
-
List of macroeconomic variables used for FLI incorporation
-
Forecasting period and reversion to long-term average
-
-
Variability in the impact and different sensitivities from FLI
-
Effect of non-linearity and probability framework
-
-
Focus on backtesting practices
-
Staging allocation
-
ECL measurement
-
IFRS 9 LGD estimates
-
IFRS 9 PD estimates
-
Overlays
-
Forward-looking information
-
CREDIT SCORING
Módulo 1: Análisis exploratorio
-
Exploratory Data Analysis EDA
-
data sources
-
Data review
-
Target definition
-
Time horizon of the target variable
-
Sampling
-
Random Sampling
-
Stratified Sampling
-
Rebalanced Sampling
-
-
Exploratory Analysis:
-
histograms
-
Q Q Plot
-
Moment analysis
-
boxplot
-
-
Treatment of Missing values
-
Multivariate Imputation Model
-
-
Advanced Outlier detection and treatment techniques
-
Univariate technique: winsorized and trimming
-
Multivariate Technique: Mahalanobis Distance
-
-
Over and Undersampling Techniques
-
Random oversampling
-
Synthetic minority oversampling technique (SMOTE)
-
Módulo 2: Feature engineering
-
Feature engineering
-
Data Standardization
-
Variable categorization
-
Equal Interval Binning
-
Equal Frequency Binning
-
Chi-Square Test
-
-
Binary coding
-
WOE Coding
-
WOE Definition
-
Univariate Analysis with Target variable
-
Variable Selection
-
Treatment of Continuous Variables
-
Treatment of Categorical Variables
-
Information Value
-
Optimization of continuous variables
-
Optimization of categorical variables
-
-
Exercise 1: EDA Exploratory Analysis
-
Exercise 2: Feauture Engineering
-
Exercise 3: Detection and treatment of Advanced Outliers
-
Exercise 4: Multivariate model of imputation of missing values
-
Exercise 5: Univariate analysis in percentiles in R
-
Exercise 6: Continuous variable optimal univariate analysis in Excel
Machine Learning
Módulo 3: Machine Learning
-
Unsupervised models
-
K Means
-
Principal Component Analysis (PCA)
-
Advanced PCA Visualization
-
Supervised Models
-
Ensemble Learning
-
Bagging trees
-
Random Forest
-
Boosting
-
Adaboost
-
Gradient Boosting Trees
-
Exercise 7: Segmentation data using K-Means
-
Exercise 8: Credit Scoring Support Vector Machine
-
Exercise 9: Credit Scoring Boosting
-
Exercise 10: Credit Scoring Bagging
-
Exercise 11: Credit Scoring Random Forest, R and Python
-
Exercise 12: Credit Scoring Gradient Boosting Trees
DEEP LEARNING
Módulo 4: Deep Learning
-
Feed Forward Neural Networks
-
Single Layer Perceptron
-
Multiple Layer Perceptron
-
Neural network architectures
-
Activation function
-
Back propagation
-
Directional derivatives
-
gradients
-
Jacobians
-
Chain rule
-
Optimization and local and global minima
-
-
Deep Learning Convolutional Neural Networks CNN
-
CNN for pictures
-
Design and architectures
-
convolution operation
-
filters
-
strider
-
padding
-
Subsampling
-
pooling
-
fully connected
-
Credit Scoring using CNN
-
Recent CNN studies applied to credit risk and scoring
-
-
Deep Learning Recurrent Neural Networks RNN
-
Long Term Short Term Memory (LSTM)
-
Hopfield
-
Bidirectional associative memory
-
descending gradient
-
Global optimization methods
-
RNN and LSTM for credit scoring
-
One-way and two-way models
-
-
Generative Adversarial Networks (GANs)
-
Generative Adversarial Networks (GANs)
-
Fundamental components of the GANs
-
GAN architectures
-
Bidirectional GAN
-
Training generative models
-
Credit Scoring using GANs
-
-
Exercise 14: Credit Scoring using Deep Learning Feed Forward
-
Exercise 15: Credit scoring using deep learning CNN
-
Exercise 16: Credit Scoring using Deep Learning LSTM
-
Exercise 17: Credit Scoring using GANs
Módulo 5: Tuning Hyperparameters in Deep Learning
-
Hyperparameterization
-
Grid search
-
Random search
-
Bayesian Optimization
-
Train test split ratio
-
Learning rate in optimization algorithms (e.g. gradient descent)
-
Selection of optimization algorithm (e.g., gradient descent, stochastic gradient descent, or Adam optimizer)
-
Activation function selection in a (nn) layer neural network (e.g. Sigmoid, ReLU, Tanh)
-
Selection of loss, cost and custom function
-
Number of hidden layers in an NN
-
Number of activation units in each layer
-
The drop-out rate in nn (dropout probability)
-
Number of iterations (epochs) in training a nn
-
Number of clusters in a clustering task
-
Kernel or filter size in convolutional layers
-
Pooling size
-
Batch size
-
Interpretation of the Shap model
-
Exercise 18: Tuning hyperparameters in Xboosting, Random forest and SVM models for credit scoring
-
Exercise 19: Tuning hyperparameters in Deep Learning model for credit scoring
Módulo 6: El proceso de desarrollo del Scorecard
-
Scoring assignment
-
Scorecard Classification
-
Scorecard WOE
-
Binary Scorecard
-
Continuous Scorecard
-
-
Scorecard Rescaling
-
Factor and Offset Analysis
-
Scorecard WOE
-
Binary Scorecard
-
-
Reject Inference Techniques
-
cut-off
-
parceling
-
Fuzzy Augmentation
-
Machine Learning
-
-
Advanced Cut Point Techniques
-
Cut-off optimization using ROC curves
-
-
Exercise 20: Creating a scorecard using Excel, R, and Python
QUANTUM COMPUTING
Módulo 7: Quantum Computing y Algoritmos
-
Future of quantum computing in banking
-
Is it necessary to know quantum mechanics?
-
QIS Hardware and Apps
-
Quantum operations
-
Qubit representation
-
Measurement
-
Overlap
-
Matrix multiplication
-
Qubit operations
-
Multiple Quantum Circuits
-
Entanglement
-
Deutsch Algorithm
-
Quantum Fourier transform and search algorithms
-
Hybrid quantum-classical algorithms
-
Quantum annealing, simulation and optimization of algorithms
-
Quantum machine learning algorithms
-
Exercise 21: Quantum operations
QUANTUM MACHINE LEARNING
Módulo 8: Desarrollo del Scorecard mediante
Quantum Machine Learning
-
What is quantum machine learning?
-
Qubit and Quantum States
-
Quantum Automatic Machine Algorithms
-
Quantum circuits
-
K means quantum
-
Support Vector Machine
-
Support Vector Quantum Machine
-
Variational quantum classifier
-
Training quantum machine learning models
-
Quantum Neural Networks
-
Quantum GAN
-
Quantum Boltzmann machines
-
Quantum machine learning in Credit Risk
-
Quantum machine learning in credit scoring
-
quantum software
-
Exercise 22: Quantum Support Vector Machine to develop credit scoring model
-
Exercise 23: Quantum feed forward Neural Networks to develop a credit scoring model and PD estimation
-
Exercise 24: Quantum Convoluted Neural Networks to develop a credit scoring model and PD estimation
Módulo 9: Tensor Networks para Machine Learning
-
What are tensor networks?
-
Quantum Entanglement
-
Tensor networks in machine learning
-
Tensor networks in unsupervised models
-
Tensor networks in SVM
-
Tensor networks in NN
-
NN tensioning
-
Application of tensor networks in credit scoring models
-
Exercise 25: Construction of credit scoring and PD using tensor networks
PROBABILISTIC MACHINE LEARNING
Módulo 10: Probabilistic Machine Learning
-
Probability
-
Gaussian models
-
Bayesian Statistics
-
Bayesian logistic regression
-
Kernel family
-
Gaussian processes
-
Gaussian processes for regression
-
-
Hidden Markov Model
-
Markov chain Monte Carlo (MCMC)
-
Metropolis Hastings algorithm
-
-
Machine Learning Probabilistic Model
-
Bayesian Boosting
-
Bayesian Neural Networks
-
Exercise 26: Gaussian process for regression
-
Exercise 27: Bayesian neural networks
VALIDACIÓN AVANZADA
Módulo 11: Validación avanzada de modelos de IA
-
Integration of state-of-the-art methods in interpretable machine learning and model diagnosis.
-
Data Pipeline
-
Feature Selection
-
Black-box Models
-
Post-hoc Explainability
-
Global Explainability
-
Local Explainability
-
Model Interpretability
-
Diagnosis: Accuracy, WeakSpot, Overfit, Reliability, Robustness, Resilience, Fairness
-
Model comparison
-
Comparative for Regression and Classification
-
Fairness Comparison
-
-
Exercise 28: Validation and diagnosis of advanced credit scoring models
IFRS 9 : EXPECTED CREDIT LOSSES
Probability of Default
Módulo 12: Probability of Default PD
-
PD estimation
-
econometric models
-
Machine Learning Models
-
Data requirement
-
Risk drivers and credit scoring criteria
-
Rating philosophy
-
Pool Treatment
-
-
PD Calibration
-
Default Definition
-
Long run average for PD
-
Technical defaults and technical default filters
-
Data requirement
-
One Year Default Rate Calculation
-
Long-Term Default Rate Calculation
-
-
PD Model Risk
-
Conservatism Margin
-
-
PD Calibration Techniques
-
Anchor Point Estimate
-
Mapping from Score to PD
-
Adjustment to the PD Economic Cycle
-
Rating Philosophy
-
PD Trough The Cycle (PD TTC) models
-
PD Point in Time PD (PD PIT ) models
-
-
-
PD Calibration of Models Using Machine and Deep Learning
-
Margin of Caution
-
Exercise 29: Modeling the Margin of Caution PD
Módulo 14: Modelos econométricos de IA de la PD
-
PD estimation
-
Treatment of Panel data
-
Econometric models to estimate PD
-
PD Logistic Regression
-
PD Probit Regression
-
PD COX regression of survival
-
PD Log-log Complementary
-
PD Regression Data Panel
-
PD Bayesian Logistic Regression
-
-
Machine Learning models to estimate PD
-
Cox XGBoost
-
Survival Tree
-
Random Survival Forest
-
Deep Learning Survival
-
PD Neural Networks
-
PD Quantum Neural Networks
-
-
PD Calibration
-
Calibration of econometric models
-
Anchor Point Estimate
-
PD calibration by vintages or vintages
-
Vintage analysis
-
PD Marginal
-
PD Forward
-
Cumulative PD
-
-
Econometric Models
-
Exercise 30: Using COX regression to estimate the PD
-
Exercise 31: Using logistic regression with panel data to estimate PD
-
Exercise 33: Using Bayesian Logistic Regression to estimate PD
-
Exercise 34: Using PD LASSO regression to estimate PD
-
Machine Learning and Quantum Machine Learning
-
Exercise 35: Using Random Forest Survival to estimate PD
-
Exercise 36: Using Cox Xboost to estimate PD
-
Exercise 37: Using Deep Learning survival to estimate PD
-
Exercise 38: Using Feed Forward NN to estimate PD
-
Exercise 39: Using Quantum Neural Networks to estimate PD
Módulo 15: Calibración de la PD
-
Concept of adjustment to central tendency
-
Bayesian approach
-
PD calibration in developed countries
-
PD calibration in emerging countries
-
Scaled PD Calibration
-
Scaled Likelihood ratio calibration
-
Smoothing of PD curves
-
Quasi moment matching
-
Approximation methods
-
Scaled beta distribution
-
Asymmetric Laplace distribution
-
-
Rubber function
-
Platt scaling
-
Broken curve model
-
Isotonic regression
-
Gaussian Process Regression
-
Exercise 40: PD calibration using Platt scaling and isotonic regression
-
Exercise 41: PD calibration using Gaussian Process Regression
-
Exercise 42: Calibration of the PD asymmetric Laplace distribution
Módulo 16: Bayesian PD and Gaussian Process
-
Bayesian and deterministic approach
-
Expert judgment
-
Prior distributions
-
Bayes' theorem
-
Posterior distributions
-
Bayesian PD Estimation
-
Markov Chain–Monte Carlo MCMC approach
-
Credibility intervals
-
Bayesian PD in practice
-
Calibration with Bayesian approach
-
Process Gaussian regression
-
Exercise 43: Logistic Model Bayesian PD in Python
-
Exercise 44: PD using MCMC in R
-
Exercise 45: PD using Process Gaussian Regression
Módulo 17: Low Default Portfolio PD (PD LDP)
-
Confidence interval approach for PD LDP
-
PD estimation without correlations
-
PD estimation with correlations
-
One-period and multi-period estimation
-
-
Bayesian PD estimation for LDP
-
Neutral Bayesian
-
Conservative Bayesian
-
expert judgment
-
-
Real analysis of PD of Corporate, Sovereign and Retail portfolios
-
LASSO regression to measure corporate default rate
-
Generating synthetic data for LDP using GAN
-
Exercise 46: PD LDP confidence interval approach in R
-
Exercise 47: Multiperiod confidence interval approach PD LDP
-
Exercise 48: Neutral Bayesian PD in R
-
Exercise 49: Conservative Bayesian PD in R
-
Exercise 50: Generating synthetic data with GAN for estimating PD
LIFETIME PD IFRS 9
Módulo 18: Matrices de transición y estructura temporal de la PD
-
Temporary structure of PD in IFRS 9
-
Properties of transition matrices
-
Markov chains
-
Multi-year transition matrix
-
discrete time
-
continuous time
-
Generating Matrix
-
Exponential of a matrix
-
-
Duration method
-
Cohort method
-
Error management
-
PD temporary structure
-
Calibration of the temporal structure of the PD
-
Levenberg–Marquardt Algorithm
-
Economic Cycles
-
Calibration of the temporal structure of the PD for LDP
-
Exercise 51: Analysis and error exercise of Transition Matrix using cohort and duration approach in Python
-
Exercise 52: Calibration of the temporal structure of the PD
Módulo 19: Modelos Lifetime PD
-
PD Lifetime consumer portfolio
-
PD Lifetime mortgage portfolio
-
PD Lifetime Wallet Credit Card
-
PD Lifetime portfolio SMEs
-
Vintage model
-
Exogenous Maturity Vintage EMV Model
-
decomposition analysis
-
Advantages and disadvantages
-
-
Basel ASRF model
-
Matrix ASRF model
-
Leveraging IRB in IFRS 9
-
Advantages and disadvantages
-
-
Regression Models
-
Logistic Multinomial Regression
-
Ordinal Probit Regression
-
-
Survival Models
-
Kaplan–Meier
-
Cox regression
-
Advantages and disadvantages
-
-
Markov models
-
Multi-State Markov Model
-
Cox Semiparametric Model
-
Advantages and disadvantages
-
-
Machine Learning Model
-
SVM: Kernel Function Definition
-
Neural Network: definition of hyperparameters and activation function
-
-
Quantum Machine Learning Models
-
PD Lifetime Extrapolation Models
-
Exercise 53: using vintage EMV Decomposition model for estimating PD Lifetime
-
Exercise 54: using multinomial regression for estimating PD Lifetime
-
Exercise 55: using Multi-State Markov Model for estimating PD Lifetime
-
Exercise 56: using matrix ASRF model for estimating PD Lifetime
-
Exercise 57: using SVM in Python for estimating PD Lifetime
-
Exercise 58: using Neural Network in Python for estimating PD Lifetime
-
Exercise 59: using Quantum Neural Network in Python for estimating PD Lifetime
-
Exercise 60: using Quantum SVM in Python for estimating PD Lifetime
Módulo 20: Calibración avanzada PD Lifetime
-
Calibration by nonlinear equation systems
-
Temporal structure calibration with Vasicek model
-
Calibration of transition matrices using Vasicek
-
Bayesian calibration
-
Fitting curve distributions
-
Extrapolation Calibration
-
Gamma Adjustment
-
exponential accelerator
-
Lifetime PD Recalibration
-
-
Nelson Siegel Calibration
-
Exercise 61: Lifetime Advanced Calibration Models PD Nelson Siegel
-
Exercise 62: Lifetime PD Advanced Calibration Models Gamma Adjustment
-
Exercise 63: Factor Fit Calibration
-
Exercise 64: Vasicek model calibration
LIFETIME PD QUANTUM
Módulo 21: Estimación de la PD Lifetime mediante
Quantum Computing
-
PD lifetime modeling
-
Exogenous Maturity Vintage
-
Age Period Cohort
-
Classic Monte Carlo simulation
-
Quantum Monte Carlo Simulation
-
Quadratic acceleration over the classical Monte Carlo simulation
-
PD lifetime modeling
-
Monte Carlo Markov Chain MCMC
-
Quantum enhancement in MCMC
-
Exercise 65: Lifetime PD IFRS 9 estimation using quantum enhancements
LGD IFRS 9
Módulo 22: LGD IRB en Retail Portfolios
-
Impact of COVID-19 on LGD
-
definition of default
-
moratoriums
-
Renovations and restructuring
-
Default Cycle
-
Real Default Cycles
-
-
Expected Loss and Unexpected Loss in the LGD
-
LGD in Default
-
Default Weighted Average LGD or Exposure-weighted average LGD
-
LGD for performing and non-performing exposures
-
Treatment of collaterals in the IRB
-
Workout approach
-
Techniques to determine the discount rate
-
Treatment of recoveries, expenses and recovery costs
-
Default Cycles
-
recovery expenses
-
-
Downturn LGD in consumer portfolios
-
Downturn LGD in Mortgages
-
LGD in consumption
-
LGD in Mortgages
-
LGD in companies
-
LGD for portfolios with replacement
Módulo 23: Modelos econométricos y de aprendizaje automático para estimar LGD
-
Advantages and disadvantages of LGD Predictive Models
-
Forward Looking models incorporating Macroeconomic variables
-
Parametric and non-parametric models and transformation regressions
-
Typology of LGD Multivariate Models
-
Linear regression and Beta transformation
-
Linear Regression and Logit Transformation
-
Linear regression and Box Cox transformation
-
Logistic and Linear Regression
-
Logistic and nonlinear regression
-
Censored Regression
-
Generalized Additive Model
-
Beta regression
-
Inflated beta regression
-
-
Support Vector Regression
-
Support Vector Classification
-
Random Forest Regression
-
XGBoosting Regression
-
Neural networks
-
Deep learning
-
Exercise 66: econometric models for estimating LGD:
-
Logistic and Linear Regression
-
Beta regression
-
-
Exercise 67: Machine Learning and Deep Learning Models for estimating LGD:
-
Random Forest Regression
-
XGBoosting Regression
-
Deep learning Regression
-
-
Exercise 68: Comparison of the performance of the models using Calibration and precision tests
Módulo 24: modelos de LGD en IFRS 9
-
Comparison of IRB LGD vs. IFRS 9
-
Impact on COVID-19
-
IFRS 9 requirements
-
Probability Weighted
-
Forward Looking
-
-
IRB LGD adjustments
-
Selection of Interest Rates
-
Allocation of Costs
-
floors
-
Treatment of collateral over time
-
Duration of COVID-19
-
-
LGD PIT modeling
-
Collateral Modeling
-
LGD IFRS 9 for portfolio companies
-
LGD IFRS 9 for mortgage portfolio
-
LGD IFRS 9 for corporate portfolios
-
credit cycle
-
Tobit Regression
-
-
IFRS 9 LGD using LASSO Regression
-
Machine Learning Models
-
Support Vector Machine
-
Neural Networks
-
-
Exercise 69: using Tobit regression in R for estimating LGD IFRS 9
-
Exercise 70: using Neural Networks regression and classification for estimating LGD IFRS 9
-
Exercise 71: using Support Vector Machine regression and classification for estimating LGD IFRS 9
EAD IFRS 9
Módulo 25: Modelización avanzada de EAD y CCF en la IFRS 9
-
Impact of COVID-19 on credit lines
-
Guidelines for estimating CCF
-
Guidelines for Estimating CCF Downturn
-
Temporal horizon
-
Transformations to model the CCF
-
Approaches to Estimating CCF
-
Fixed Horizon approach
-
Cohort Approach
-
Variable focus time horizon
-
-
Econometric Models
-
Beta regression
-
Inflated beta regression
-
Fractional Response Regression
-
Mixed Effect Model
-
-
Machine Learning Models
-
neural networks
-
SVM
-
-
Intensity model to measure the withdrawal of credit lines
-
Exercise 72: using OLS Regression Model for estimating CCF
-
Exercise 73: using CCF Logistic Regression Model for estimating CCF
-
Exercise 74: using Neural Networks and SVM for estimating CCF
EAD IFRS 9
Módulo 26: Modelización de la tasa de prepago
-
Prepaid and other options
-
IFRS 9 requirements
-
Probability Weighted
-
Forward Looking
-
IFRS 9 prepayment modeling
-
Cox regression
-
Logistic regression
-
-
Survival rate estimate
-
Joint Probability Model with Prepayment rate and Lifetime PD
-
Exercise 75: Modeling IFRS 9 prepayment model for mortgage portfolios in R and Excel
Módulo 27: Lifetime EAD para líneas de crédito
-
Impact of the pandemic on the use of credit lines
-
Lifetime measurement in credit cards
-
Lifetime EAD
-
IFRS 9 requirements
-
Probability Weighted
-
Forward Looking
-
Adjustments in the EAD
-
Interest Accrual
-
CCF PIT Estimate
-
Lifetime CCF Estimate
-
Lifetime EAD modeling
-
Model of the use of credit lines with macroeconomic variables
-
Credit card abandonment adjustment
-
Lifetime EADmodel for pool of credit lines
-
vintage model
-
Chain Ladder Approach
-
-
Exercise 73: Econometric model of credit line usage in R
-
Exercise 74: Lifetime EAD model for individual line of credit
-
Exercise 75: Vintage Lifetime EAD Model for Pool of Credit Lines in R and Excel
STRESS TESTING ECL
Módulo 28: Preparación de modelos econométricos
-
Review assumptions of econometric models
-
Review the coefficients and standard errors of the models
-
Model reliability measures
-
Error management
-
Normal test
-
Heteroscedasticity
-
Outliers
-
Autocorrelation
-
Using Correlation to detect bivariate collinearity
-
Identifying the existence of multivariate collinearity in linear regression models.
- Identifying the presence of multivariate collinearity in logistic regression.
-
Exercise 76: Detecting non-stationary series, identifying cointegration, and detecting outliers.
-
Exercise 77: Measurement of collinearity,
-
Exercise 78: Measurement of heteroskedasticity
-
Exercise 79: Measuring Serial Autocorrelation
AI STRESS TESTING
Módulo 29: Deep Learning para modelizar la Dinámica macroeconómica
-
Macroeconomic models
-
Neoclassical growth model
-
Partial differential equations
-
DSGE Stochastic Dynamic General Equilibrium Models
-
Deep learning architectures
-
Reinforcement Learning
-
Advanced Scenario Analysis
-
Exercise 80: using neural networks for Bellman equation macroeconomic model
STRESS TESTING PD and LGD
Módulo 30: Forecasting y Stress Testing PD y LGD usando AI
-
Forecasting models
-
Multivariate Models
-
VAR Autoregressive Vector Models
-
ARCH models
-
GARCH models
-
GARCH Models Multivariate Copulas
-
VEC Error Correction Vector Model
-
Johansen's method
-
-
Machine Learning Models
-
Supported Vector Machine
-
Neural network
-
Multivariate Adaptive Regression Splines
-
Development and validation base
-
-
Deep learning
-
Recurrent Neural Networks RNN
-
Elman's Neural Network
-
Jordan Neural Network
-
Basic structure of RNN
-
Long short term memory LSTM
-
temporary windows
-
Development and validation sample
-
Regression
-
Sequence modeling
-
Machine Learning learning and Quantum Machine Learning
-
Exercise 81: Forecasting PD using Random Forest
-
Exercise 82: Forecasting PD using Neural Networks
-
Exercise 83: Forecasting PD using LSTM
-
Exercise 84: Forecasting PD using QUANTUM LSTM
Probabilistic Machine Learning
-
Exercise 85: Forecasting PD using Gaussian Process
-
Exercise 86: Forecasting PD using Bayesian Additive Regression Trees
-
Exercise 87: Forecasting PD using Bayesian Support Vector Machine
-
Exercise 88: Forecasting PD using Bayesian Neural Networks
-
Exercise 89: Forecasting PD using Pandemic variables and climate- change using RNN LSTM in Python
-
Exercise 90: Charge-off model using RNN LSTM
Módulo 31: Stress Testing Tradicional de PD y LGD
-
Temporal horizon
-
Multi-period approach
-
Data required
-
Impact on P&L, RWA and Capital
-
Machine Learning Models
-
Probabilistic Machine Learning Models
-
Macroeconomic Stress Scenarios in consumption
-
Expert
-
Statistical
-
regulatory
-
-
Stress Testing PD :
-
Credit Portfolio View
-
Multiyear Approach
-
Reverse Stress Testing
-
Rescaling
-
Cox regression
-
-
Stress Testing of the Transition Matrix
-
Approach Credit Portfolio View
-
credit cycle index
-
Multifactor Extension
-
-
LGD Stress Testing:
-
LGD Downturn: Mixed Distribution Approach
-
PD/LGD Multiyear Approach modeling
-
LGD stress test for mortgage portfolios
-
-
Stress Testing of:
-
Defaults
-
Charge Off
-
Net Charge Off
-
Roll Rates
-
Rating/Scoring transition matrices
-
Delinquency bucket transition matrices
-
Recovery Rate and LGD
-
Losses on new impaired assets
-
Losses on old impaired assets
-
-
Exercise 91: Stress Testing PD in Excel and SAS multifactorial model Credit Portfolio Views
-
Exercise 92: Stress Testing PD in SAS Multiyear Approach
-
Exercise 93: Stress test of PD and Autoregressive Vectors
-
Exercise 94: Stress Test LGD
-
Exercise 95: Joint Stress Test of the PD and LGD
-
Exercise 96: Charge-off model using VAR and VEC
Módulo 32: Stress Testing en portfolios corporate
-
Temporal horizon
-
Data required
-
Main Macroeconomic variables
-
Impact on P&L, RWA and Capital
-
ASRF model
-
Creditmetrics model
-
Using Transition Matrices
-
Use of the credit cycle index
-
Default forecasting
-
Stress Test Methodology for corporate portfolios
-
Impact on RWA and Capital
-
Exercise 97: Stress Testing PD and corporate portfolio transition matrices using transition matrix and ASRF model in SAS, R and Excel
STRESS TESTING ECL IFRS 9
Módulo 33: Stress Testing bajo ECL IFRS 9
-
Stress testing under IFRS 9 and COVID-19
-
Pandemic scenarios applied to the ECL calculation
-
Stress Testing of IFRS 9 parameters
-
EBA Stress Testing 2023
-
Treatment of the moratorium
-
Possible regulatory scenarios
-
Impact on P&L
-
PIT starting parameters
-
PIT projected parameters
-
Calculation of non-productive assets and impairments
-
Changes in the stock of provisions
-
Changes in the stock of provisions for exposures phase S1
-
Changes in the stock of provisions for exposures phase S2
-
Changes in the stock of provisions of exposures phase S3
-
Sovereign Exposure Impairment Losses
-
Impact on capital
-
Internal Stress Testing Model for ECL IFRS 9
-
Stage Migration
-
Stage Transition Matrix
-
Stress testing of PDs and credit migrations
-
Stress testing of exhibitions
-
Stress testing of recoveries
-
Exercise 98: Internal global exercise of ECL Stress Testing in R and Excel
QUANTUM COMPUTING PARA STRESS TESTING Y
SIMULACIÓN DE MONTE CARLO
Módulo 34: Quantum Computing para el Stress Testing
-
Quantum economics
-
Classic Monte Carlo simulation
-
Quantum Monte Carlo
-
Coding Monte Carlo problem
-
Breadth Estimation
-
Acceleration applying the amplitude estimation algorithm
-
DGSE model using neural networks
-
Quantum Monte Carlo Simulation vs Normal Monte Carlo Simulation
-
Exercise 95: DGSE model using deep learning
-
Exercise 96: Quantum Monte Carlo Simulation vs. Classical Monte Carlo Simulation ECL in Stress Testing
Aumento significativo del riesgo de crédito
SICR in IFRS 9
Módulo 35: Significant Increase in Credit Risk SICR
-
Significant increase in credit risk (SICR)
-
Impact of COVID-19 on the increase in SICR risk
-
Recommendations Basel, EBA, ESMA, IFRS
-
Qualitative and quantitative criteria based on COVID-19
-
Increase in collective credit risk
-
Individual IFRS 9 credit risk increase
-
Phase migration matrices
-
Roll rate models
-
Markov model
-
-
Impact of COVID-19 on migrations
-
Estimation of PD Lifetime and PD Origination thresholds
-
Rating Variation
-
Determination of thresholds
-
KRIs for retail, mortgages and corporate
-
Increase in collective IFRS 9 credit risk
-
Use of discriminant test
-
ROC curve
-
false alarm rate
-
target hit rate
-
S2 size
-
-
Exercise 97: Estimating SICR credit risk increase using ROC discriminant power test in R and Excel
IFRS 9 ECL MODEL
Módulo 36: Modelos de estimación de las pérdidas crediticias esperadas ECL
-
Macroeconomic scenarios impacted by COVID-19
-
Lifetime Loss Forecasting using macroeconomic variables
-
Global Exercise 98: Estimating Lifetime Expected Credit Losses for a Consumer Credit Portfolio using R, Excel, and VBA.
-
Definition of macroeconomic scenarios COVID-19
-
Impact of the scenarios on the estimate for COVID-19
-
LGD modeling using economic scenarios
-
CCF modeling using economic scenarios
-
Abandonment Modeling
-
Prepayment Modeling
-
PD PIT modeling with economic scenarios
-
Lifetime PD Modeling
-
Estimate of financial income
-
Cash flow modeling
-
Estimated survival rate
-
12-month ECL Expected Loss Estimate
-
12-month ECL estimate COVID-19 effect
-
ECL Lifetime Expected Loss Estimate IFRS 9 COVID-19
-
Stress Testing of credit risk losses
-
Assignment analysis of the 3 stages
-
Comparison of ECL Estimates
-
Interpretation of results in the scorecard
IFRS 9
Validación del Expected Credit Loss
Módulo 37: Validación del ECL
-
Initial validation
-
Periodic validation
-
Monitoring
-
Main milestones of qualitative validation
-
Data quality
-
Default Definition
-
Relevance of the qualification process
-
Override Analysis
-
environmental dynamics
-
user test
-
-
Main milestones of quantitative validation
-
Samples used for validation purposes
-
Discriminating Power
-
population stability
-
Characteristic Stability
-
concentration analysis
-
Staging analysis
-
Parameter Calibration
-
ECL backtesting
-
-
Principle 5 – Validation
-
Exercise 99: Global Validation of ECL IFRS 9
GENERATIVE AI EN ECL IFRS 9
Módulo 38: Generative AI
-
Introducing generative AI
-
What is Generative AI?
-
Generative AI Models
-
Generative Pre- trained Transformer (GPT)
-
-
Text generation, Image generation, Music generation, Video generation
-
Generating text
-
Generating Code
-
Ability to solve logic problems
-
Generating Music
-
Enterprise Use Cases for Generative AI
-
Overview of Large Language Models (LLMs)
-
Transformer Architecture
-
Types of LLMs
-
Open-Source vs. Commercial LLMs
-
Key Concepts of LLMs
-
-
Prompts
-
Tokens
-
Embeddings
-
Model configuration
-
Prompt Engineering
-
Model adaptation
-
Emergent Behavior
-
Specifying multiple Dataframes to ChatGPT
-
Debugging ChatGPT’s code
Human errors -
Exercise 100: Embeddings for words, sentences, question answers
-
Exercise 101: Embedding Visualization
-
Exercise 102: First let's prepare the data for visualization
-
Exercise 103: PCA (Principal Component Analysis)
-
Exercise 104: Embeddings on Large Dataset
-
Exercise 105: Prompt engineering
-
Exercise 106: Advanced Prompting Techniques
-
Exercise 107: Large Language Models (LLMs)
GENERATIVE AI en ECL IFRS 9
-
Exercise 108: Modeling Lifetime PD using generative AI
-
Exercise 109: Using Transformers for forecasting Lifetime PD
-
Exercise 110: Analysis of ECL results using generative AI
-
Exercise 111: Applications of autoregresive LLM in ECL IFRS 9